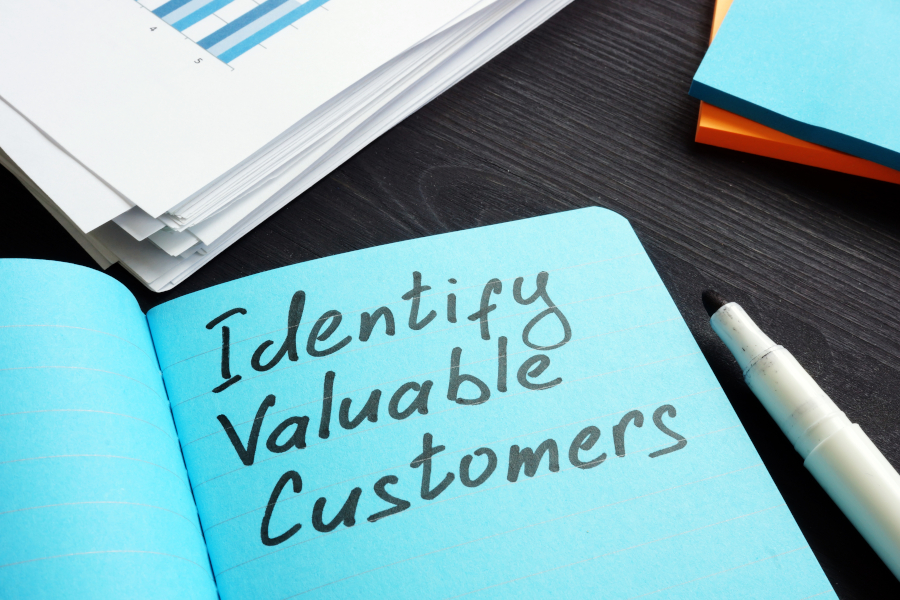
Personalization activities for even more effective customer engagement-oriented marketing strategies: let’s talk again about the RFM matrix, one of the most effective audience profiling and segmentation models in eCommerce.
In a previous article we had the opportunity to delve into this model from a theoretical point of view, understanding its potential compared to more traditional profiling and segmentation models. Unlike the latter, in fact, the RFM matrix model takes into consideration historical and behavioural data of the entire audience, and is not based on general demographic data, nor on representative samples. Precisely by virtue of this nature, such a profiling and segmentation model allows one to get to know one’s customers in greater depth and enables one to identify the most interesting clusters on which to deploy effective ring-fencing strategies aimed at increasing their Customer Lifetime Value.
- Who are my best customers?
- Which customers are potentially more “likely” to buy at a higher price?
- Which customers can be retained?
- Which ones, however, are most likely not to repurchase in our shop?
These are just some of the questions that a careful analysis of one’s audience conducted through an RFM matrix allows us to answer. But let’s find out, in detail, some of the clusters that can be identified and the strategies we can deploy for each of these.
RFM matrix: advanced profiling and segmentation
As we have already discussed, the RFM model is based on three different variables:
- recency (recency): indicates the number of days since the customer’s last purchase;
- frequency: identifies the number of orders placed in a given time period, usually a year;
- monetary (monetary value): refers to the total amount spent by customer in a given time frame, again the year is taken as a reference.
The data collected relating to the three variables above is then categorised using a scoring system that allows the RFM model to be created to all intents and purposes. At this stage, the adoption of a Customer Data Platform, such as Blendee, allows the potential of artificial intelligence to be exploited not only to collect and analyse data, but also to process it through the adoption of a scoring system.
The result? An evolved profiling and segmentation of its audience using the RFM model. Here then are some identifiable clusters:
- customers who spend a lot, have bought recently, and buy frequently (high recency, high monetary, high frequency);
- customers who purchase frequently, have purchased recently but do not spend much (high frequency, high recency, low monetary);
- customers that we cannot lose because they have purchased so much in the past (high frequency, high monetary, low recency);
- promising customers who have recently purchased, spent a lot, but still have not purchased often (high recency, high monetary, low frequency);
In addition to the most promising clusters, such as those shown above, we can identify others that instead require activities aimed at final ringing:
- customers who have not purchased for a long time, who may have made one purchase and spent little;
- customers who have purchased often in the past, perhaps on promotions, have not spent much and have not purchased for some time;
- lost customers i.e. those who have the lowest values in terms of frequency, recency and amount spent.
RFM matrix and customization: for each segment, the right strategy!
Once the different user segments and clusters have been identified, fielding the most effective strategy and providing for different levels of personalization of the customer experience will be even easier and more straightforward:
- high-spending, recent and frequent shoppers may be the best ambassadors for a brand and could be hired for new product launches;
- the most loyal customers who buy often, even if they do not spend much, can be stimulated to buy more valuable products perhaps with personalized product recommendations;
- customers who have not purchased for a long time, but have purchased a lot in the past, could be ringaged with surveys to get feedback and ad hoc promotions;
- customers who are more responsive to offers could be ringed with product proposals, even at higher prices, but still in line with their recent purchases;
- to customers instead who have the lowest values in terms of frequency, monetary, and recency we could send periodic offers.
In the era of personalization of the customer experience, knowing one’s audience thoroughly is the first step in deploying successful marketing strategies. The RFM matrix represents, without a shadow of a doubt, one of the most effective models at this juncture as it allows one to empirically identify the clusters of users on whom it is most interesting to work and allows one to focus on the most effective strategy to engage them.