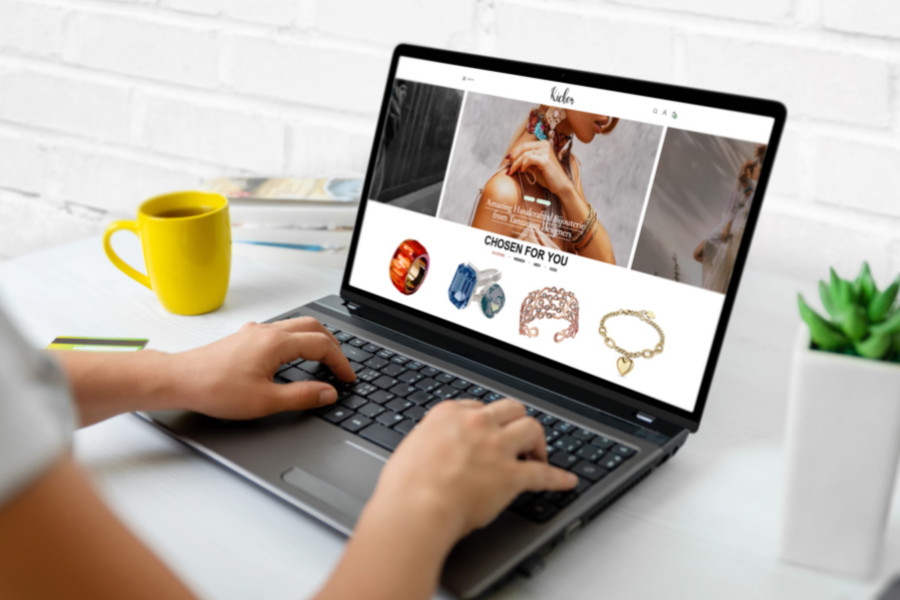
It is 1994 when Jeff Bezos abandons a secure professional position in a hedge found and, in the basement of his own rented house, founds Amazon. In its first month of life, the eCommerce sells books in every U.S. state and in more than 45 different countries around the world, generating a net profit of $20,000 per week, the rest is history.
Behind Amazon’s success, apart from its revolutionary business model, lies a constant improvement of the platform and the continuous search for innovative solutions. Among these, we cannot forget the product recommendation engine, the dynamic product recommendation ‘engine’ that has made Amazon’s success and revolutionised the entire eCommerce world.
We have chosen, thus, to open today’s article of our blog, precisely by recalling this story. According to a study released by McKinsey, up to 35 percent of Amazon sales are generated thanks to the artificial intelligence algorithms that govern product recommendations. Jeff Wilke, director of the Consumer Division, thus comments on the role of this feature in the world’s best-known eCommerce platform:
At Amazon.com, we use recommendation algorithms to personalize the online store for each customer. The store changes radically based on customer interests, showing programming books to an engineer and baby toys to a new mom.
Product recommendation: but what if my eCommerce is not Amazon?
As can be easily guessed, at Amazon nothing is left to chance, but everything is governed by the artificial intelligence and by machine learning. The engine that governs dynamic product recommendations is composed of several parts that deal with the analysis of multiple data: it basically selects products to show to each user based on purchases made, interactions and ratings of items on display, and combines the results with similar items viewed by users with similar interests.
Although of Amazon and eCommerce platforms with so much expendable budget in research and development there is only one, dynamic product/content recommendation features can also be implemented on smaller eCommerce (and why not content sites), thanks to marketing automation solutions and platform such as Blendee, with undoubted advantages:
- increased sales;
- improving users’ shopping and browsing experience;
- increased customer retention;
- customer retention.
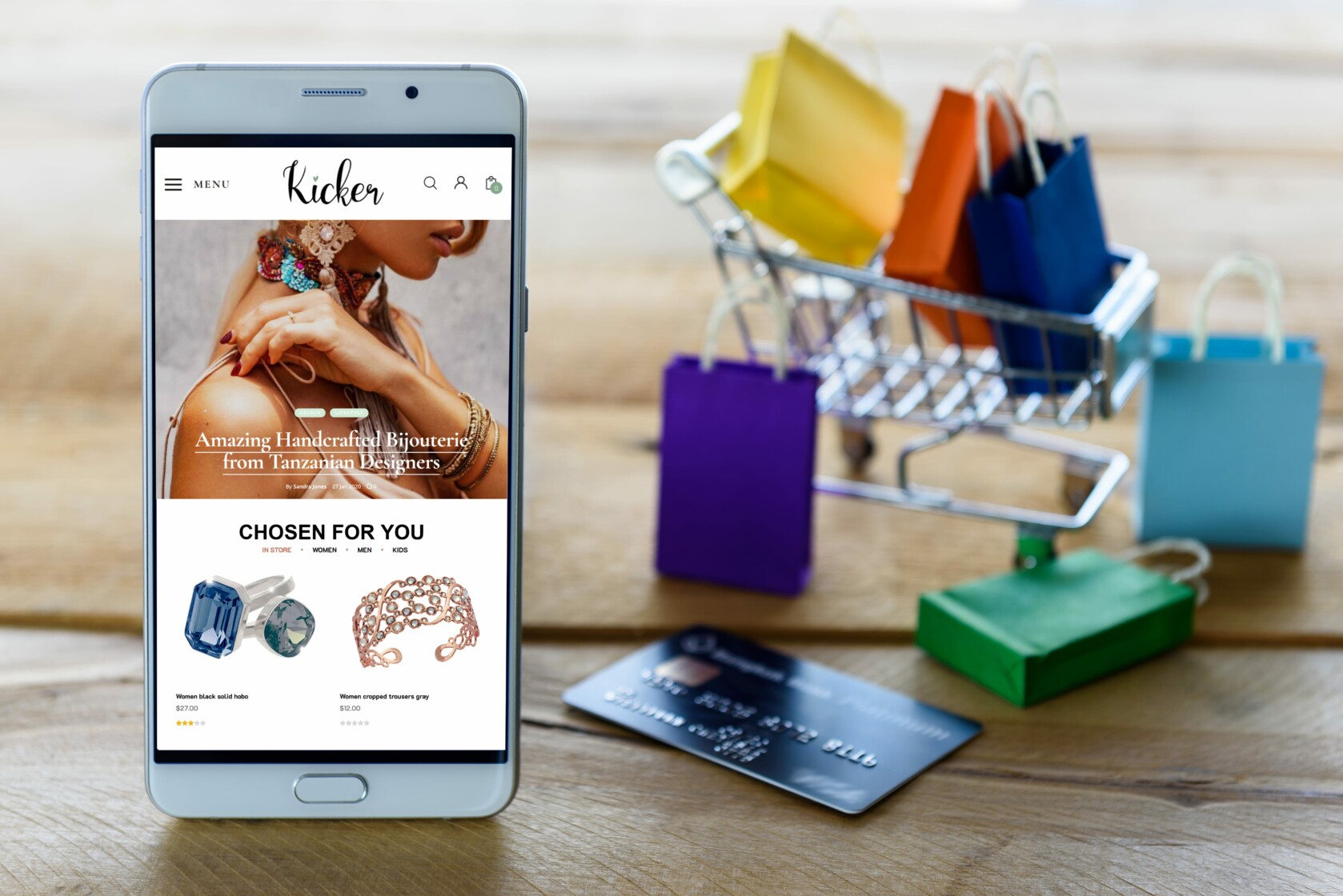
Product Recommendation with Blendee: how to do it?
It is estimated, on average, that only 5 percent of catalog products are actually seen by users-this is when the use of dynamic product recommendations becomes critical not only to let users know about your catalog products but to show them at the most opportune time.
Profiling and segmenting the audience upstream will be critical to selecting the most appropriate cluster of users to whom to show certain product recommendations. But let’s go in order.
Blendee allows the ability to configure product recommendations based on multiple algorithms. Here are the main ones:
- trending recommendation: the most popular products in the catalog are shown by click and view;
- personalized recommendation: selected products are suggested to the user based on his or her recent browsing history within the site;
- personalized trending recommendation: in this case the algorithm shows products by mixing the previous two;
- browsing history recommendation: products are shown from those that the user has viewed;
- personalized recommendation by sales: the algorithm allows showing suggested products from those that the user has recently purchased;
- shopping cart recommendation: products are recommended from those that the user has placed in the shopping cart;
- remarketing recommendation: products that the user has viewed in the last few days but has not purchased are shown.
Choosing the algorithm but not only that: in the configuration phase, it is also essential to select the page of the site where you want to show them:
- home page;
- category page;
- product page;
- blog page;
- account page;
- page 404;
- cart page.
Site/eCommerce page blocks but not only: Blendee also allows you to insert dynamic product recommendations within behavioral messages (warnings, popups..) and within emails and newsletters (email widgets).
Dynamic product recommendations: how to choose the right algorithm?
Among so many available algorithms, how can they choose the winning one? This is definitely the question you are asking yourself.
There are guidelines, pretty much established, that based on the page in question and the cluster of users you want to intercept there are some algorithms that tend to be more effective than others.
Home page For first-time users, it is recommended to select generic algorithms to show trending products, while to returning users it is preferable to show recommended products based on their browsing or purchasing path.
Product page In this case, the goal of showing recommended products is to raise the value of the receipt and stimulate the user to purchase a product similar to the one they are viewing at a higher price.
Shopping cart page It is recommended to show cross-selling recommended products, i.e., products that are complementary to those that the user has added to the shopping cart, taking advantage of the dedicated algorithm itself (shopping cart recommendation).
Category page Again, it is possible to show at the top of the listing the most viewed and clicked on products in the particular category that the user is viewing.
Page 404 and search results In this case, it is possible to show the most viewed and clicked products from the user’s browsing history.
The above are only best practices: with in each situation only data analysis can confirm the greater or lesser effectiveness of one algorithm over another.
Effective product recommendation? Blendee is the one to tell you which one to choose!
Thanks to Experiments, the new A/B testing version of Blendee, choosing the most effective product recommendation is even easier.
In a few simple clicks, you can compare two or more product recommendation algorithms in a given section of your site or email, taking just a cluster of your users as your target audience.
You can choose whether to set up the test in automatic or manual mode. In the first case you will only need to choose according to which criterion to select the winning variant (views, clicks, sales), while in the second case you will also need to choose the degree of accuracy of the test, the number of users to be involved, the time duration and the waiting time before data analysis.
Once two or more algorithms have been tested and the winner identified, this will be used as a criterion to orchestrate the display of dynamic recommended products.